How Machine Learning Could Transform Work in Physics Labs Across the Country
Recently published research from Bradley Fugetta (C’23), Gen Yin and collaborators could transform the way physics labs around the world study the magnetic properties of different materials by using machine learning.
“Magnets are useful across a range of applications,” said Fugetta, the paper’s lead author and a postbaccalaureate fellow in the Department of Physics. “Even if you’re not interested in physics, being able to more fully understand the magnetic properties of different materials will allow for more useful and innovative electronics and technologies.”
Published in Physical Review Research, the paper proposes using machine learning to estimate magnetic material parameters from experimental measurements that are relatively simple, cheap and already commonplace in magnetics labs worldwide.
The results could carry more subtle information that is currently only available through costly, time-intensive research.
Mining Into Magnets
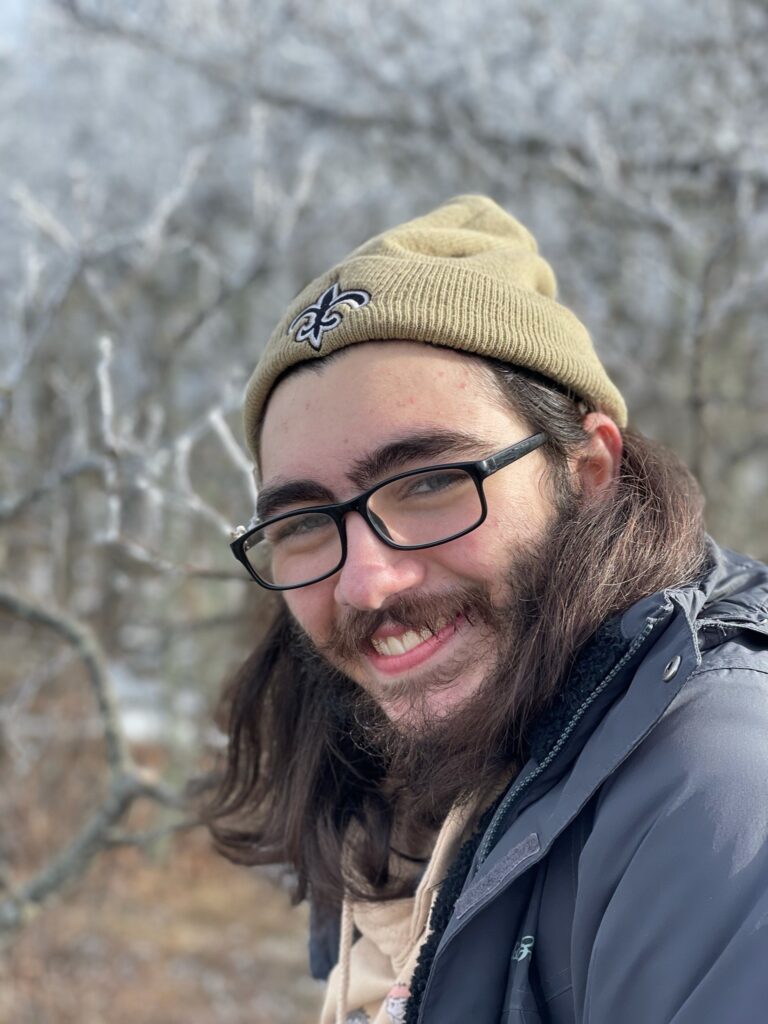
Bradley Fugetta (C’23), the paper’s lead author.
All magnetic materials possess intrinsic properties that differ from one another and dictate how they behave. Understanding how those properties operate individually and interact in concert can help scientists and engineers predict a material’s viability for different use cases, whether it’s upgrading your laptop or your refrigerator.
“Everyone from physicists at universities to the product designers at large tech companies wants to better understand how different magnetic materials function,” said Fugetta. “Unfortunately, doing an in-depth analysis of a material requires expensive equipment and a lot of time – two things that not every physics lab has in great quantities.”
Fugetta, who graduated from the College of Arts & Sciences last year with a major in physics and minors in math and classics, began conducting research under Yin, an assistant professor in the Department of Physics, as a sophomore. Yin, who initially conceived of the research project and its parameters, acted as Fugetta’s main advisor to the project.
“Magnets are actually made up of trillions and trillions of tiny magnetic particles, forming structures which we call spin textures,” said Fugetta. “By mapping out these spins, which are invisible to the human eye, physicists can predict how magnetic materials with any given properties will behave in a range of scenarios.”
Working with fellow graduate students at Georgetown and several notable physicists, including Kai Liu and Amy Liu, Fugetta began exploring how machine learning could be used to predict the strength of a certain nanoscale interaction from large-scale experimental measurements. The analysis presents what the research team calls “magnetic fingerprints” of different materials.
Fugetta is a member of Phi Beta Kappa, the oldest academic honor society in the country, and Theta Alpha Kappa, the national religious studies and theology honors society. Last year, Fugetta received the Kidwell Medal, which is awarded to the graduating senior who has demonstrated the highest level of academic achievement in physics coursework, as well as the Undergraduate Research Award for his work on this project.
-by Hayden Frye (C’17)